Dear HeartLogic™ users,
With the continuous spread of COVID-19 in many countries, congresses have still been either cancelled or transformed into virtual sessions. This was the case of the recent ESC Congress 2020 as well, which was replaced by an online event.
As you are most probably aware, the ESC Congress Programme Committee created a digital platform to disseminate the latest and most important findings in cardiovascular science. Late-Breaking Trials sessions, accepted Abstracts, Key Opinion Leader Lessons and all news from ESC were recorded and are available for registered participants to the ESC Congress 2020 – The Digital Experience until September 30th.
Remote heart failure management using a multiparameter implantable cardioverter-defibrillator alert: the multicentric RE-HEART registry
(De Juan Baguda et al.) – LIVE SESSION
The analysis presented by Dr. De Juan Baguda has been accepted by the Congress Scientific Board as an oral presentation in a Live Session.
This abstract comes from the Spanish Multicentric HeartLogicTM Registry (RE-HEART), an independent Registry that involves 17 HeartLogic centers in Spain.
The design of the study included three different phases:
- Blinded phase: analysis of correlation between clinical events occurred and HeartLogic value in a period when physicians were blinded to the HeartLogic index.
- Prospective phase in clinical practice: use of HeartLogic in clinical practice without a standardised protocol
- Prospective phase in clinical practice: use of HeartLogic in clinical practice with a standardised management protocol (still ongoing).
Phase 1

The remaining 18 alerts were unexplained (not associated with overt HF events or other clinically meaningful events), however their rate was low: 0.25 alert-patient/year.
The median time spent in alert was longer in the case of HF hospitalisations than of in-office visits.
The maximum HeartLogic index value was 38±15 in the case of hospitalisations and 24±7 in that of minor HF events (Figure 1).
Phase 2

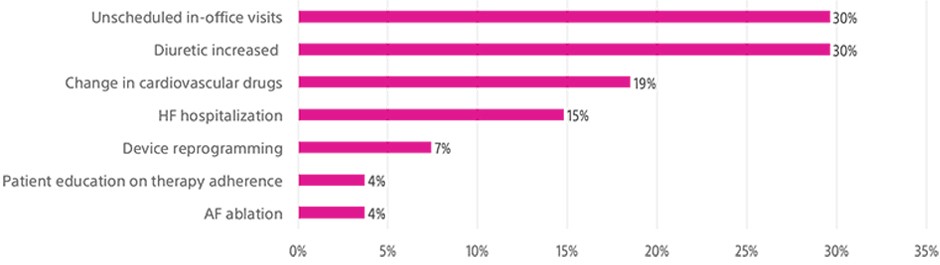
HeartLogic contributing sensors detected changes mainly in heart sound amplitude, as third sound increase and first sound decrease, than in other sensors*
- Heart Sounds: 97% of total alerts
- Thoracic Impedance: 23% of total alerts
- Respiratory Rate: 14% of total alerts
- Night Heart Rate: 6% of total alerts
*More than one sensors contributes to a single alert
Unexplained alerts:
- The rate of unexplained alerts, not followed by any clinical action, was 0.13 alert-patient/year.
- These alerts were managed remotely, through device data review and phone contact, except for only one alert that generated an unscheduled in-office visit
Conclusions
These analyses confirm the association between HeartLogic alerts and HF-related clinical events.
In blinded phase
- HeartLogic alerts were associated not only with HF hospitalisations, but also with minor events of HF decompensation or changes in prescribed HF therapy.
- Minor events resulted in shorter duration of the alert state and lower index value
In active phase:
- Relevant clinical conditions were remotely detected using HeartLogic alert
- The adoption of the alerting system allowed to remotely implement clinical actions
- Among the sensed parameters that contribute to the calculation of the index, accelerometer-based heart sounds seemed to be the most sensitive
Remote heart failure management using A multiparametric ICD algorithm for heart failure risk stratification: an analysis in clinical practice
(Prof. L. Calò) – POSTER SESSION
This analysis is intended to evaluate the risk stratification ability of the HeartLogic algorithm in a group of patients who received the ICD and CRT-D devices, equipped with HeartLogic, in clinical practice.
The HeartLogic feature was activated in 257 patients with the baseline characteristics reported in Table 1
Parameters | N=257 |
Age, years | 70±11 |
Male, n (%) | 186 (72 |
LVEF, % | 30±8 |
Ischemic Cardiomyopathy, n (%) | 127 (49) |
Valvular disease, n (%) | 60 (23) |
Chronic Kidney Disease, n (%) | 93 (36) |
COPD, n (%) | 47 (18) |
Diabetes, n (%) | 77 (30) |
Hypertension, n (%) | 163 (63) |
History of AF, n (%) | 108 (42) |
Patients were followed for a median follow-up of 14 months [25-75 percentile: 7-19] and the following events occurred:
- 40 HF hospitalisations requiring at least 1 overnight stay (0.14/patient-year)
- 191 HeartLogic index crossing (threshold set at 16) in 105 patients.
The time in the alert state was 27 patient-years, i.e. 9.5% of the total observation period.
HF hospitalisation rate was
- 0.96/patient-year while in alert state
- 0.05/patient-year while out of alert state.
The occurrence of ≥1 index crossing during follow-up was associated with the risk of HF hospitalisation independently from other baseline clinical variables (Table 2).
| Logistic regression model | ||
| OR | 95% CI | P |
Age | 0,9754 | 0,9387 to 1,0135 | 0,203 |
Male | 0,6266 | 0,2421 to 1,6216 | 0,335 |
LVEF | 1,0001 | 0,9723 to 1,0286 | 0,997 |
Ischemic Cardiomyopathy | 0,7692 | 0,3244 to 1,8239 | 0,551 |
Chronic Kidney Disease | 1,6992 | 0,7182 to 4,0199 | 0,228 |
COPD | 0,9351 | 0,3027 to 2,8886 | 0,907 |
NYHA Class | 0,9476 | 0,4726 to 1,9002 | 0,880 |
Diabetes | 1,9173 | 0,8018 to 4,5847 | 0,143 |
Hypertension | 1,3528 | 0,5354 to 3,4181 | 0,523 |
History AF | 1,2947 | 0,5487 to 3,0549 | 0,555 |
HL Crossing ≥1 | 4,7008 | 1,7863 to 12,3704 | <0,002 |
Conclusions
This analysis of data collected in clinical practice confirms that the multiparametric ICD algorithm is an independent predictor of higher risk of HF.
In particular, it allows dynamic identification of time-intervals when patients are at significantly increased risk of worsening HF. This potentially helps better triage resources to a more vulnerable patient population.